Arm Tech Predictions for 2025 and Beyond
At Arm, we are constantly thinking about the future of computing. From the latest architectural features to new technologies for silicon solutions, everything we create and design is geared towards how technology will be used and experienced in the future.
This is supported by our unique position in the technology ecosystem where we have a vast understanding of the global, interconnected and highly specialized semiconductor supply chain covering all markets, from IoT to datacenters and everything in between. This means we have a broad range of insights into the future direction of technology and key trends that are likely to emerge in the years ahead.
With this in mind, we have the following technology predictions for 2025 and beyond, covering all aspects of technology, from the future growth of AI to silicon designs to key trends across different technology markets. Read on to see what Arm thinks the year ahead will bring…
Silicon
A rethinking of silicon design with chiplets being part of this solution
From a cost and physics perspective, it is getting increasingly difficult to do traditional silicon tape-outs. The industry will need to rethink silicon designs and go beyond these traditional approaches. For example, there is a growing realization that not everything needs to be integrated on a single, monolithic chip, with new approaches like chiplets beginning to emerge as foundry and packaging companies find new ways to push the boundaries of Moore’s law, but under new dimensions.
The different implementation techniques of chiplets are getting more attention and having a deep impact on core architecture and micro-architecture. For chiplets, architects will need to be increasingly aware of what different implementations offer, whether it’s the manufacturing process node or the packaging technology, and then take advantage of the features for performance and efficiency benefits.
Chiplets are already addressing specific market needs and challenges, with this likely to advance in the years ahead. In the automotive market, chiplets can help companies achieve auto-grade qualifications during the silicon development process They can also help to scale and differentiate silicon solutions through utilizing different computing components. For example, chiplets focused on compute have a different number of cores, whereas memory-focused chiplets have different sizes and types of memories, so by combining and packaging these various chipsets at a system integrator level companies can develop a greater number of highly differentiated products.
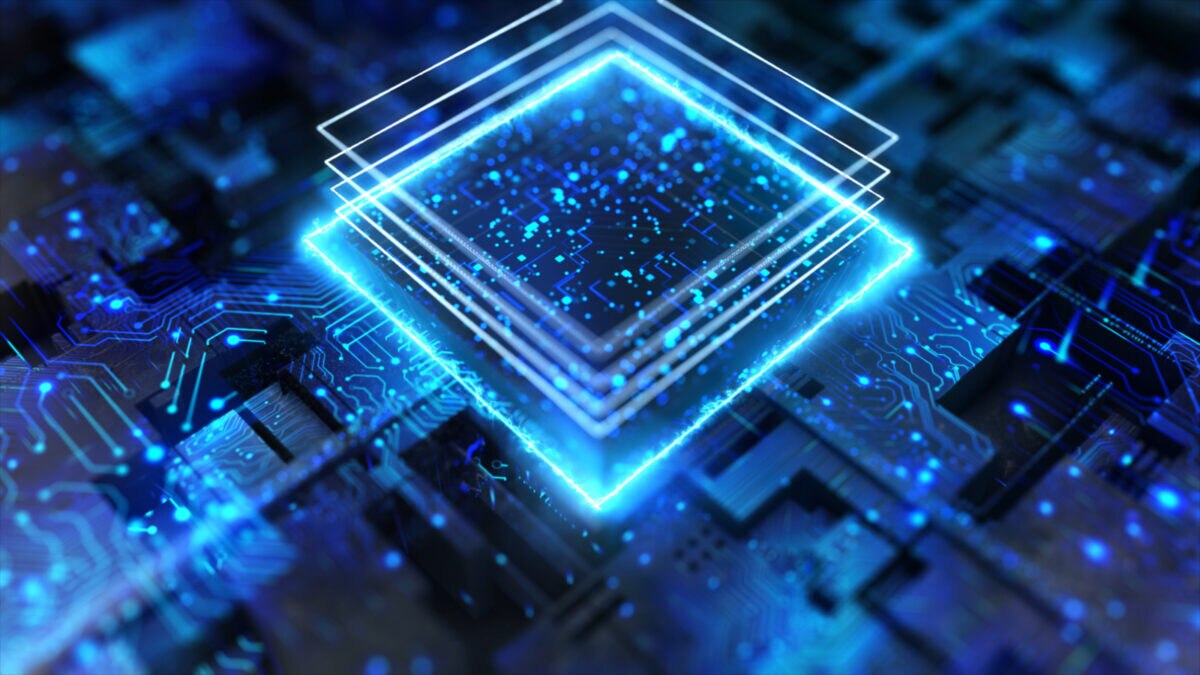
The Moore’s Law “recalibration”
Moore’s Law has put billions of transistors on a chip, doubled performance and halved power every year. However, this continuous push for more transistors, more performance and less power on a single, monolithic chip is not sustainable. The semiconductor industry will need to rethink and recalibrate Moore’s Law and what it means to them.
Part of this means moving away from solely focusing on performance as the key metric and instead valuing performance per watt, performance per area, performance per power and total cost of ownership as core metrics during silicon design. There are also new metrics that focus on the implementation aspect of the system – which present the most challenges for development teams – and making sure performance is not degraded once the IP is integrated into a system-on-chip (SoC) and then the overall system. Therefore, this will involve continuous performance optimizations during silicon development and deployment. These metrics are more relevant to where the wider tech industry is heading, as it pushes for more efficient computing for AI workloads.
The growth of specialized silicon
The industry-wide push for specialized silicon will continue to grow. The rise of AI has put power consumption in focus, emphasizing that the datacenter can no longer be built around off-the-shelf computing solutions. Instead, the compute must be built and designed around specific datacenters and workloads. Across the industry, we have witnessed a movement towards custom silicon, particularly with leading cloud hyperscalers, including Amazon Web Services (AWS), Google Cloud and Microsoft Azure. In 2025, we expect this movement to continue with significant investment in technologies that allows leading technology companies to more rapidly design and deploy custom silicon, from ASIC services to chiplets.
True commercial differentiation in silicon solutions
The push for more specialized silicon will be part of the ongoing move for companies to deliver true commercial differentiation with their silicon solutions. A big part of this is the growing adoption of compute subsystems (CSS), which are core computing components that enable companies – big and small – to differentiate and customize their solutions, with each configured to perform or contribute towards specific computing functions or specialized functionalities.
The growing importance of standardization
Standardized platforms and frameworks are vital to ensure the ecosystem can differentiate their products and services, adding true commercial value while saving time and costs. With the emergence of chiplets that integrate different computing components, standards will be more important than ever, as it will enable different hardware from different vendors to work together seamlessly. Arm is already working with more than 50 technology partners on the Arm Chiplet System Architecture (CSA) and expect more to join as part of this growing push towards standardization in the chiplet marketplace. In the automotive industry, this will be combined with SOAFEE that aims to de-couple hardware and software in software-defined vehicles (SDVs), leading to greater flexibility and interoperability between computing components and faster development cycles.

Ecosystem collaboration on silicon and software like we have never seen before
As the complexities of silicon and software continue to grow, no single company will be able to cover every level of silicon and software design, development and integration alone, with deep levels of ecosystem collaboration needed. This provides unique opportunities for different companies – big and small – to deliver different computing components and solutions based on their core competencies. This is especially relevant to the automotive industry, which needs to bring together the entire supply chain – from silicon vendors and Tier 1s to OEMs and software vendors – to share their expertise, technologies and products to define the future of AI-enabled SDVs and fulfil its true potential for the end-user.
The rise of AI-enhanced hardware design
The semiconductor industry will see increased adoption of AI-assisted chip design tools, where AI helps optimize floor plans, power distribution, and timing closure. This approach will not only optimize performance results, but accelerate the development cycle of optimized silicon solutions and enable smaller companies to enter the market with specialized chips. While AI will not replace human engineers, it will become an essential tool in handling the growing complexity of modern chip design, particularly for power-efficient AI accelerators and edge devices.
AI
Significant investments into performant, power-efficient AI
A topic at the forefront of everyone’s minds – governments, industry and society in general – is how to manage the increasing power and compute demands in the age of AI. Finding ways to limit the power consumption of large datacenters without compromising performance is paramount, especially with the world’s datacenters requiring 460 terawatt-hours (TWh) of electricity annually, which is the equivalent to the entire county of Germany.
Performant, power efficient AI will be achieved through co-design of the entire system, with investments in both hardware and software. From a hardware perspective, further advancements in underlying processor technologies and CPU architecture will ensure that AI is processed as efficiently as possible. Specialized hardware will leverage these advancements to efficiently handle intensive AI workloads across all aspects of the datacenter, including network, storage, security, and data management. Meanwhile, new innovative software will optimize AI workloads, so they can operate with fewer resources while maintaining or improving performance.
The continuous growth of AI inference
In the year ahead, AI inference workloads will continue to grow, helping to ensure that AI can be deployed widely and sustainably everywhere. This growth is being driven by the increasing number of AI-enabled devices and services. In fact, the majority of AI inference, which covers everyday AI use cases like text generation and summarization, can take place on smartphones and laptops, which provides more responsive and secure AI-based experiences for the end-user. In order to see this growth, devices must be built upon a bedrock which enables faster processing, lower latency and efficient power management. Two key Armv9 architecture features are SVE2 and SME2, which together combine to enable fast and efficient AI workloads on the Arm CPU.
The power of heterogenous computing in the AI era
It is clear that no individual piece of hardware or computing component will be the answer for all workloads. This is especially important as AI inference continues to permeate all aspects of compute across all types of devices, from smart thermostats to the datacenter. We have seen significant growth of AI accelerators in 2024 including from leading hyperscalers, but to leverage those accelerators for AI workloads requires a CPU platform. Arm Neoverse is providing a level of flexible computing that makes the coupling of an Arm CPU with an accelerator seamless, enabling new types of engineering creativity and development like we have seen with the Grace Blackwell superchip that pairs NVIDIA’s Blackwell GPU architecture with the Arm Neoverse-based Grace CPU. The Arm CPU is the most ubiquitous compute platform in the world, and we expect to see more of these heterogenous compute collaborations in 2025.

AI at the edge will grow in prominence
In 2024, we have seen an increasing number of AI workloads running at the edge – on device – rather than being processed in large datacenters. This means power and cost savings, as well as privacy and security benefits for consumers and businesses.
2025 will also likely see the emergence of sophisticated hybrid AI architectures that separate AI tasks between edge devices and the cloud. These systems will use AI algorithms in edge devices to detect events of interest before employing cloud models to provide additional information. Determining where to run AI workloads, locally or in the cloud, will be based on factors like available power, latency requirements, privacy concerns, and computational complexity.
Edge AI workloads represent a shift towards decentralized AI, enabling smarter, faster, and more secure processing on the devices closest to the data source, which will be particularly beneficial in markets that require higher performance and localized decision-making, such as industrial IoT and smart cities.
The acceleration of smaller language models (SLMs)
Smaller, compact models with an increased compression, quantization and a decreasing number of parameters are evolving at a rapid rate. Examples include Llama, Gemma and Phi3, which are more cost-effective, efficient, and easier to deploy on devices with limited computational resources and we expect the number to grow in 2025. These models can run directly on edge devices, bringing enhanced performance and privacy.
We expect to see a rise in SLMs used for on-device tasks for language and device interactions, as well as vision-based tasks, such as interpreting and scanning for events. In the future, learnings will be distilled from larger models to develop local expert systems.
Multimodal AI models that can hear, see and understand more
Right now, large language models (LLMs), like GPT-4, are trained on human text. If these models are asked to describe a scene, they respond with a text-based description. However, we are beginning to see the advent of multimodal AI models that include text, images, audio, sensor data and many more. These multimodal models will deliver more advanced AI-based tasks through audio models that can hear, vision models that can see, and behavior models that can understand relationships between people and objects. These will give AI the ability to sense the world just as humans do – being able to hear, see and experience.
The growing use of AI agents
If users interact with AI today, they are likely to be interacting with a single AI that will do its best to complete the task requested on its own. With AI agents, a person still tells one AI that task required, but it would delegate this to a network of AI agents or bots. Examples of industries that have started using AI agents today include customer service support and coding assistants. We expect this to grow substantially in the year ahead across more industries, as AI becomes even more connected and intelligent. This will help to set the next stage for the AI revolution, leading to an even bigger impact on productivity for both our personal and work lives.
More powerful, intuitive, intelligent applications
Fueled by the rise of AI, there will be more powerful and personalized applications on devices. These will include more intelligent and intuitive personal assistants, and even personal physicians, with applications moving away from simply reacting to user requests, to making proactive suggestions based on the user and the environment they find themselves in. The move towards the hyper-personalization of AI through such applications will lead to an exponential rise in data usage, processing and storage, which supports the need for greater security measures and regulatory guidance from industry and governments.
Healthcare will be the key AI use case
Healthcare appears to be one of the leading use cases for AI, with this set to accelerate in 2025. Examples of AI for health use cases include predictive healthcare, digital record storage, digital pathology, the development of vaccines and gene therapy to help cure diseases. In 2024, the founders of DeepMind were awarded the Nobel Prize for Chemistry, as they worked with scientists to use AI to predict complex protein structures with 90 percent accuracy. Meanwhile, the use of AI has been shown to shorten the R&D cycle during the drug research process by 50 percent. The benefits of such AI innovations to society are significant, accelerating the research and creation of life-saving medicines. Furthermore, the combination of mobile devices, sensors and AI will allow users to have access to far better health data, so they can make more informed decisions about their own personal health.
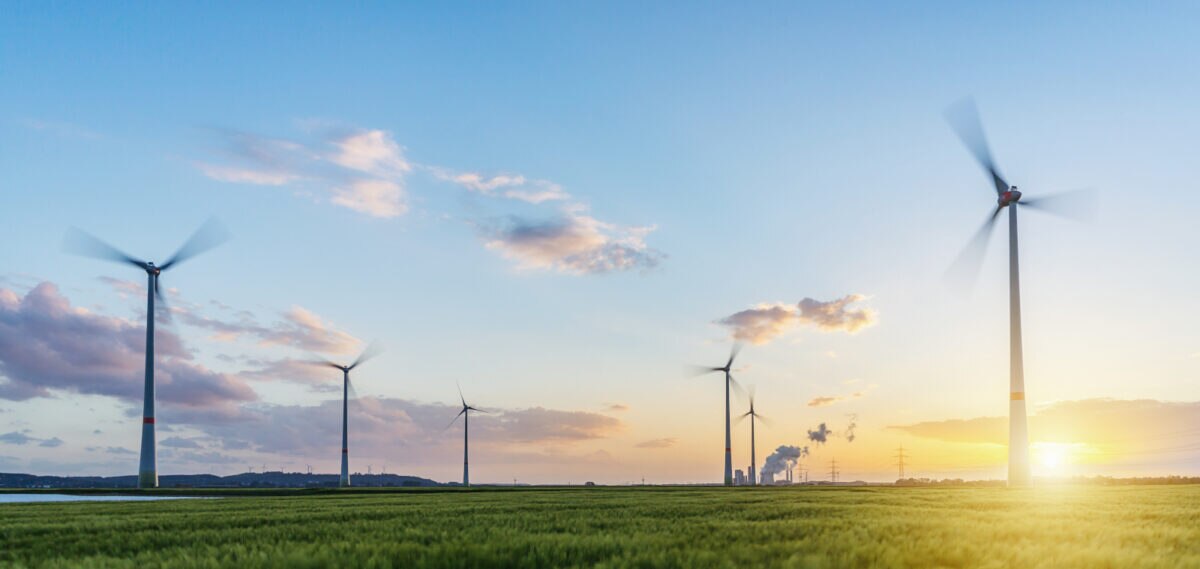
A push towards “greener AI”
The integration of sustainable practices within AI is set to accelerate. Alongside the use of power-efficient technologies, there could be an increasing focus on “greener AI” approaches. For example, in response to increasing energy demands, training AI models in lower-emission geographies and during periods of lower grid demand could evolve to become standard practice. By balancing energy loads on the grid, this approach will help mitigate peak demand stress and reduce overall emissions. Therefore, expect to see more cloud providers offering scheduling options for energy-efficient model training.
Other approaches could include optimizing existing AI models for efficiency, reusing or repurposing pre-trained AI models, and an uptake in “green coding” to minimize energy usage. We may also start to see the introduction of voluntary, then formal, standards for sustainable AI development as part of this wider move towards “greener AI.”
Advancements in renewable energy paired with AI
The combination of renewable energy and AI is expected to increase innovation across the energy sector. The reliability of renewable sources and lack of flexibility to balance peak loads currently limits energy grid decarbonization. However, we expect AI to help predict energy demand with greater accuracy, optimize grid operations in real-time, and enhance the efficiency of renewable energy sources to help tackle this. Energy storage solutions are also benefiting from AI, which optimizes battery performance and longevity that are crucial for balancing the intermittent nature of renewable energy sources.
AI integration not only offers solutions to the challenge of predicting and balancing peak demand, but also predicting maintenance. This reduces disruptions while smart grids leverage AI for real-time energy flow management and reduction of wastage. The advancements in AI paired with renewable energy promises significant improvements in efficiency and sustainability of energy systems.
Markets
Heterogeneous computing for all types of AI in IoT
The broad range of AI applications, particularly in IoT, will need to use different computational engines for different AI demands. To maximize the deployment of AI workloads, CPUs will remain a key focus for deployment on existing devices. New IoT devices will offer enhanced performance for AI with increased memory sizes and higher performance Cortex-A CPUs. Embedded accelerators, such as the latest Ethos-U NPUs, will be used to accelerate low power machine learning (ML) tasks, and bring power-efficient edge inference to a broader range of use cases such as industrial machine vision and consumer robotics.
Essentially, in the short term, we will see multiple compute elements used to serve the AI needs of specific applications. This trend will continue to emphasize the need for common tools, libraries and frameworks to enable application developers to make the most of the capabilities in the underlying hardware. There is no “one size fits-all” solution for edge AI workloads, highlighting the importance of a flexible compute platform for the ecosystem.
Increasing adoption of virtual prototypes to transform silicon and software development process (Automotive)
Virtual prototypes are accelerating silicon and software development cycles, with companies able to develop and test software before the physical silicon is ready. The benefits are particularly relevant to the automotive industry where the availability of virtual platforms is accelerating automotive development cycles by up to two years.
In 2025, we are expecting more companies to launch their own virtual platforms as part of this ongoing transformation of the silicon and software development process. These virtual platforms will work seamlessly, with Arm architecture offering ISA parity, ensuring uniformity in architecture in the cloud and at the edge. With ISA parity, the ecosystem can build their virtual prototype in the cloud and then seamlessly deploy at the edge.
This saves significant time and costs, while giving developers more time to extract even greater performance from their software solutions. Following the introduction of the Armv9 architecture to automotive markets for the very first time in 2024, we expect to see more developers take advantage of this ISA parity in automotive and leverage virtual prototyping to build and deploy automotive solutions quicker.
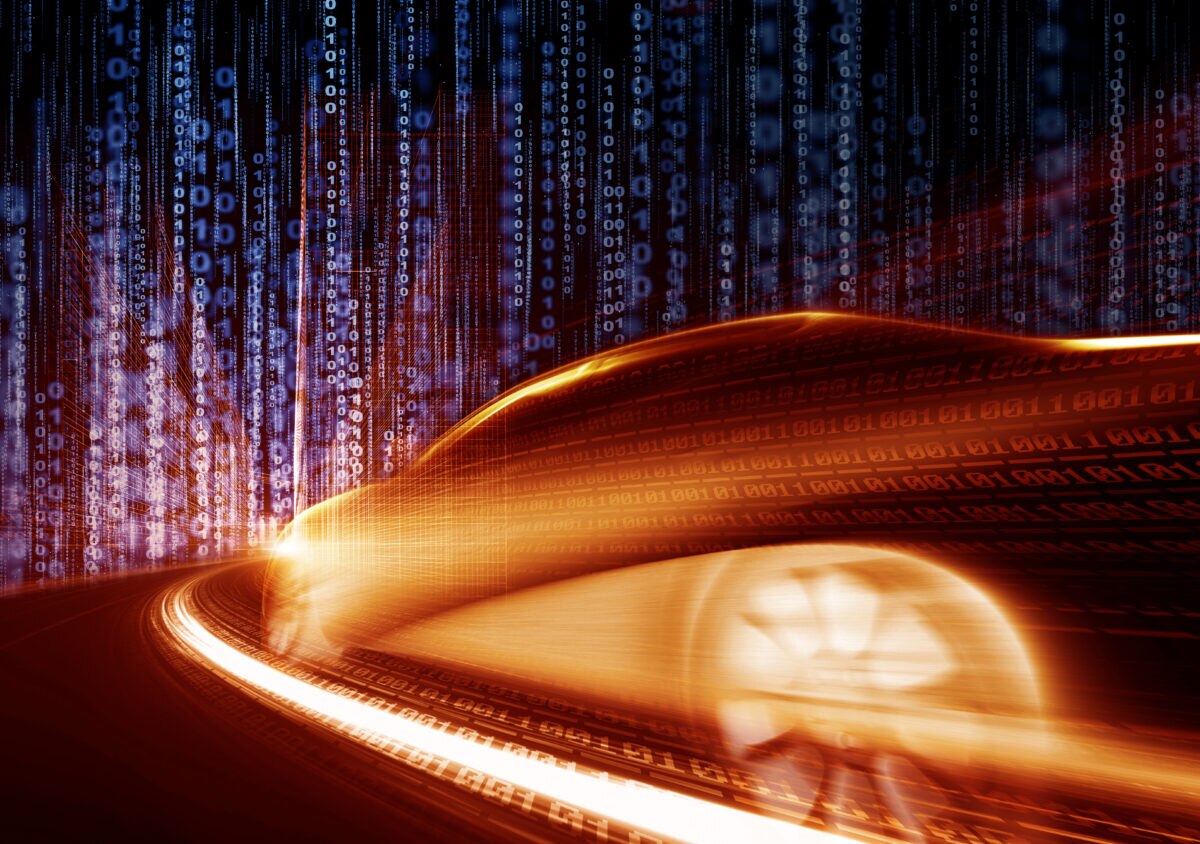
End-to-end AI will enhance automated driving systems
Generative AI technology is rapidly being adopted in end-to-end models that promise to address scalability barriers faced by traditional automated driving (AD) software architectures. With end-to-end self-supervised learning, AD systems are more capable of generalizing to cope with previously unseen scenarios. This novel approach promises an effective way of enabling faster scaling of operational design domains, making it quicker and cheaper to deploy AD technology from the highway to urban areas.
More hands-off driving, but more driver monitoring too
Progress in the harmonization of vehicle regulations for L2+ hands-off DCAS and L3 ALKS will accelerate wide deployment of these premium features worldwide. Leading automakers are already investing in equipping vehicles with the hardware necessary to up-sell these features through subscriptions throughout the lifetime of the vehicle.
In order to prevent driver misuse of driving automation systems, regulations and New Car Assessment Programs are focusing on increasingly sophisticated in-cabin monitoring systems like driver monitoring (DMS). In Europe, for example, EuroNCAP 2026’s new rating scheme will incentivize deeper integration of direct-sensing (e.g. camera-based) DMS with advanced driver assistance systems (ADAS) and AD features to provide adequate vehicle responses to different levels of driver disengagement.
The smartphone is the primary consumer device for decades, not just years
The smartphone’s crown as the world’s primary consumer device will not be going away anytime soon. In fact, it is likely to be the go-to device for consumers for decades, not just years, with no device likely to offer a realistic challenge. With adoption of Armv9 growing across leading smartphones, this will mean more computing capabilities and better application experiences from new flagship smartphones in 2025 that will only reinforce this number one position. However, it is clear that consumers use different devices for different purposes, with the smartphone being primarily used for app use, web browsing and communication, whereas PCs and laptops are still seen as the “go-to” devices for productivity and work-based tasks.
It will also be interesting to see the emergence of AR wearables, like smart glasses, as the ideal companion device for the smartphone. A key reason behind the staying power of the smartphone is its ability to evolve, from apps to cameras to gaming, and now the industry is seeing the emergence of new usage models for AR, with the smartphone beginning to support AR-based experiences from wearable devices.
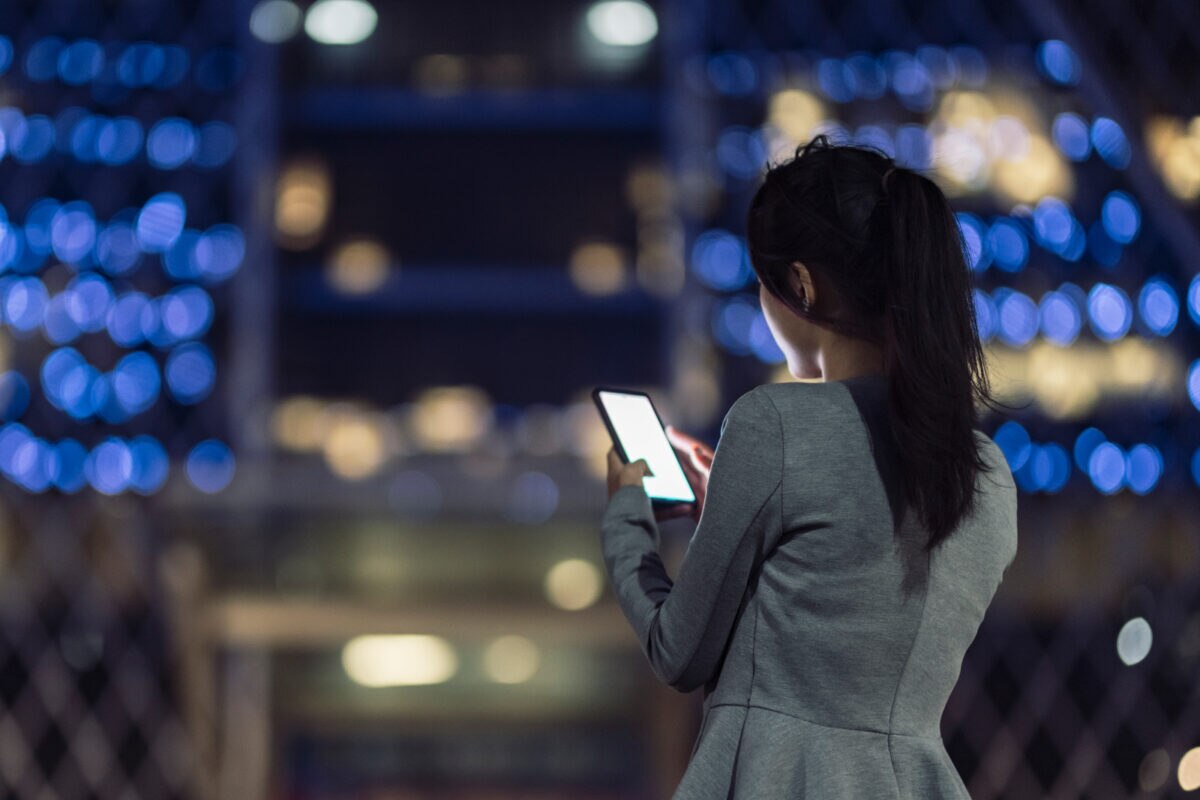
The ongoing miniaturization of technology
Across the tech industry, we are seeing smaller, sleeker devices, like AR smart glasses and smaller wearable tech. This is being made possible through a combination of factors. Firstly, the adoption of power-efficient technologies that offer the performance required to support key device features and experiences. Secondly, in the case of AR smart glasses, we are now seeing the adoption of ultra-thin silicon carbide technologies that not only enable high-definition displays, but also dramatically reduce the thickness and weight of the devices. Finally, new compact language models are transforming AI-based experiences across these smaller devices, making them more immersive and interactive. The powerful combination of power-efficiency, lightweight hardware and smaller AI models will be a driver for the growth of smaller, more capable consumer devices in the next year.
The continuous rise of Windows on Arm
In 2024, the Windows on Arm (WoA) ecosystem saw significant progress, with the most widely used applications now providing Arm-native versions. In fact, 90 percent of the time spent by the average Windows user will be with applications that are now Arm-native. One recent example is Google Drive, which released an Arm-native version at the end of 2024. We expect this momentum to continue throughout 2025 and lead to WoA becoming even more appealing for both developers and consumers, as demonstrated by the impressive performance enhancements for Arm-native applications, like Google Chrome, that are essential to everyday user experiences.
The post Arm Tech Predictions for 2025 and Beyond appeared first on Arm Newsroom.