WatchThis: A Wearable Point-and-Ask Interface Powered by Vision-Language Models and XIAO ESP32S3 Sense
MIT Media Lab researchers Cathy Mengying Fang, Patrick Chwalek, Quincy Kuang, and Pattie Maes have developed WatchThis, a groundbreaking wearable device that enables natural language interactions with real-world objects through simple pointing gestures. Cathy conceived the idea for WatchThis during a one-day hackathon in Shenzhen, organized as part of MIT Media Lab’s “Research at Scale” initiative. Organized by Cedric Honnet and hosted by Southern University of Science and Technology and Seeed Studio, the hackathon provided the perfect setting to prototype this innovative device using components from the Seeed Studio XIAO ESP32S3 suite. By integrating Vision-Language Models (VLMs) with a compact wrist-worn device, WatchThis allows users to ask questions about their surroundings in real-time, making contextual queries as intuitive as pointing and asking.
Credit: Cathy Fang
Hardwares
The WatchThis project utilizes the following hardware components:
- Seeed Studio XIAO ESP32S3 Sense (with camera expansion board)
- Seeed Studio round display for XIAO
- OV2640 camera with long ribbon cable
- LiPo Battery
- Custom 3D printed parts
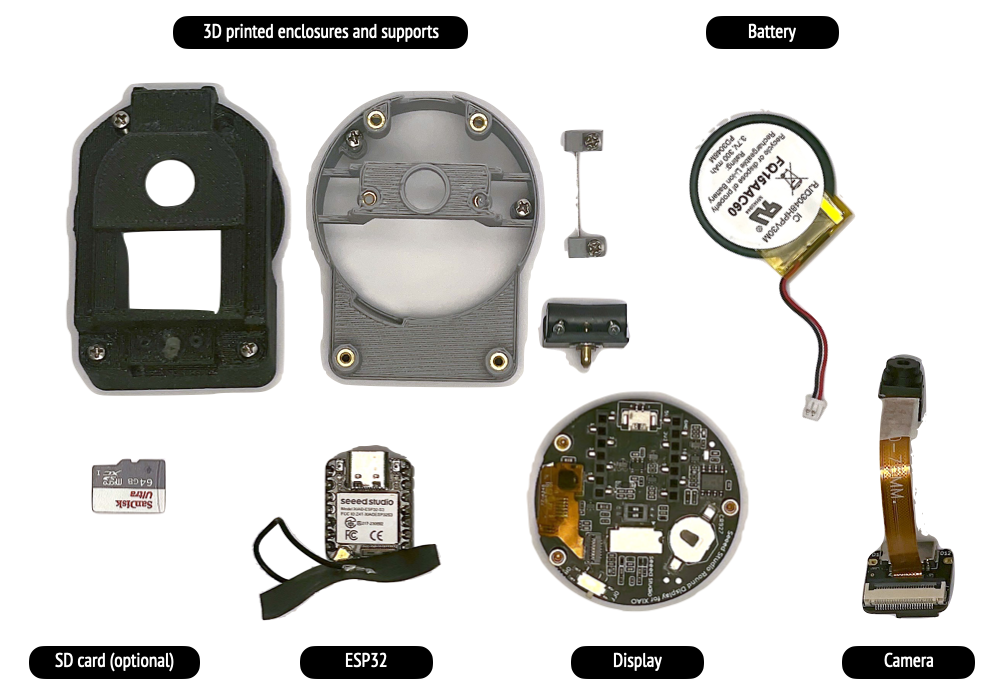
How the Project Works
WatchThis is designed to seamlessly integrate natural, gesture-based interaction into daily life. The wearable device consists of a watch with a rotating, flip-up camera attached to the back of a display. When the user points at an object of interest, the camera captures the area, and the device processes contextual queries based on the user’s gesture.
The interaction begins when the user flips up the watch body to reveal the camera, which then captures the area where the finger points at. The watch’s display shows a live feed from the camera, allowing precise aiming. When the user touches the screen, the device captures the image and pauses the camera feed. The captured RGB image is then compressed into JPG format and converted to base64, after which an API request is made to query the image.
The device uses these API calls to interact with OpenAI’s GPT-4o model, which accepts both text and image inputs. This allows the user to ask questions such as “What is this?” or “Translate this,” and receive immediate responses. The text response is displayed on the screen, overlaid on the captured image. After the response is shown for 3 seconds, the screen returns to streaming the camera feed, ready for the next command.
The software driving WatchThis is written in Arduino-compatible C++ and runs directly on the device. It is optimized for quick and efficient performance, with an end-to-end response time of around 3 seconds. Instead of relying on voice recognition or text-to-speech—which can be error-prone and resource-intensive—the system uses direct text input for queries. Users can further personalize their interactions by modifying the default query prompt through an accompanying WebApp served on the device, allowing tailored actions such as identifying objects, translating text, or requesting instructions.
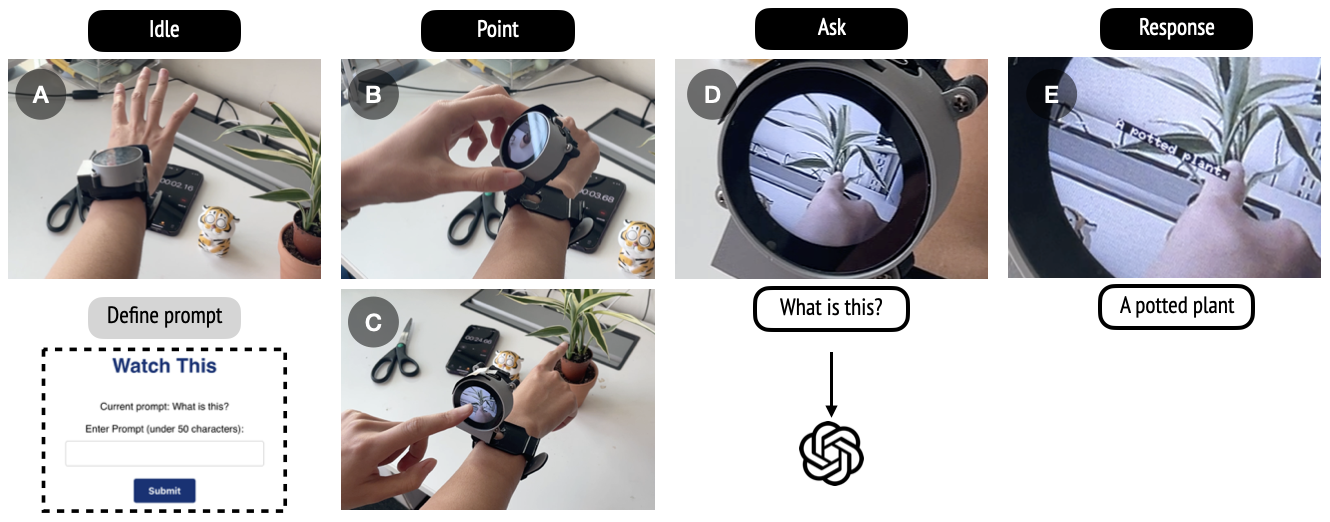
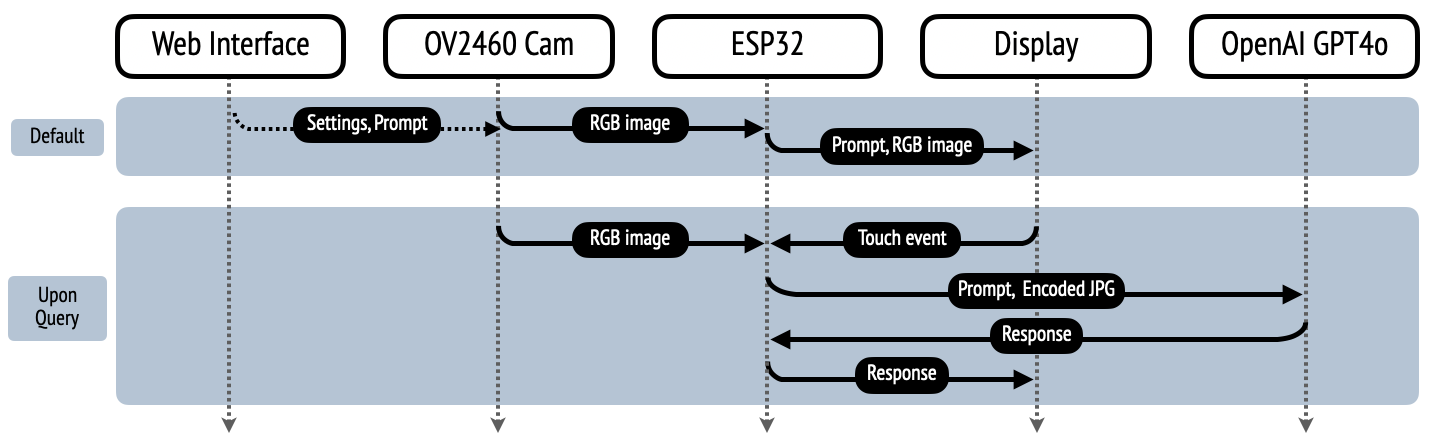
Applications
Imagine strolling through a city and pointing at a building to learn its history, or identifying an exotic plant in a botanical garden with a mere gesture.
The device goes beyond simple identification, offering practical applications like real-time translation of, for example, menu items, which is a game-changer for travelers and language learners alike.
The research team has discussed even more exciting potential applications:
- A “Remember this” function could serve as a visual reminder system, potentially aiding those who need to take medication regularly.
- For urban explorers, a “How do I get there” feature could provide intuitive, spatially-aware navigation by allowing users to point at distant landmarks.
- A “Zoom in on that” capability could offer a closer look at far-off objects without disrupting the user’s activities.
- Perhaps most intriguingly, a “Turn that off” function could allow users to control smart home devices with a combination of voice commands and gestures, seamlessly integrating with IoT ecosystems.
While some of these features are still in conceptual stages, they paint a picture of a future where our interactions with the world around us are more intuitive, informative, and effortless than ever before.
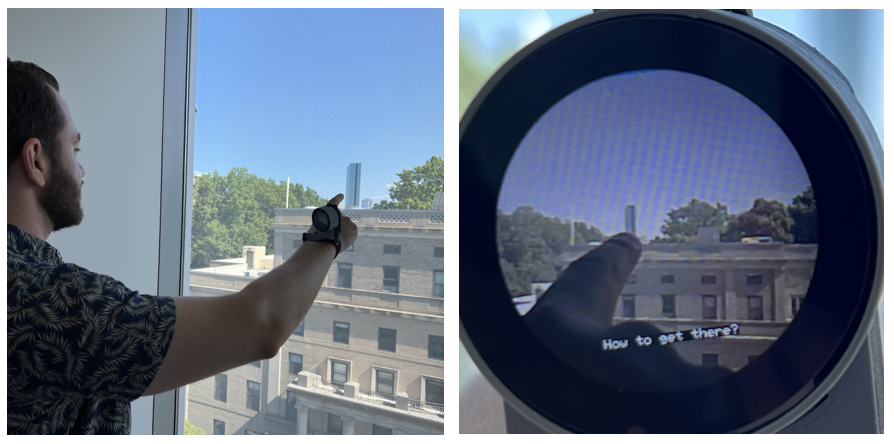
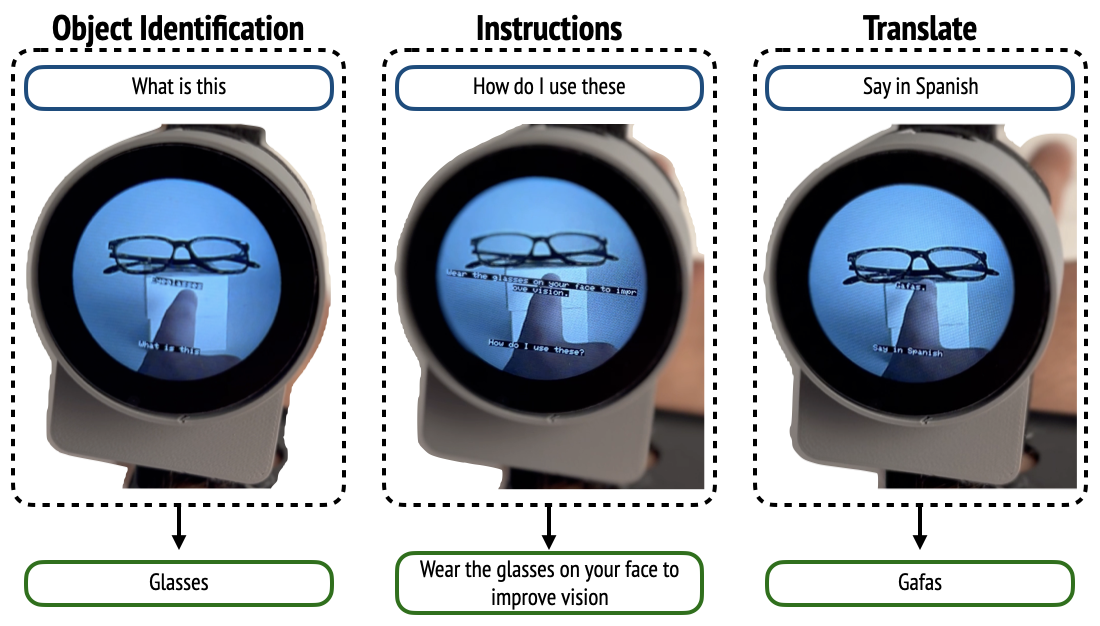
Build Your Own WatchThis
Interested in building your own WatchThis wearable? Explore the open-source hardware and software components on GitHub and start creating today! Check out their paper below for full details.
End Note
Hey community, we’re curating a monthly newsletter centering around the beloved Seeed Studio XIAO. If you want to stay up-to-date with:
Cool Projects from the Community to get inspiration and tutorials
Product Updates: firmware update, new product spoiler
Wiki Updates: new wikis + wiki contribution
News: events, contests, and other community stuff
Please click the image below to subscribe now!
The post WatchThis: A Wearable Point-and-Ask Interface Powered by Vision-Language Models and XIAO ESP32S3 Sense appeared first on Latest Open Tech From Seeed.